Prof.Sunyoung Shin
최고관리자
2024-07-11
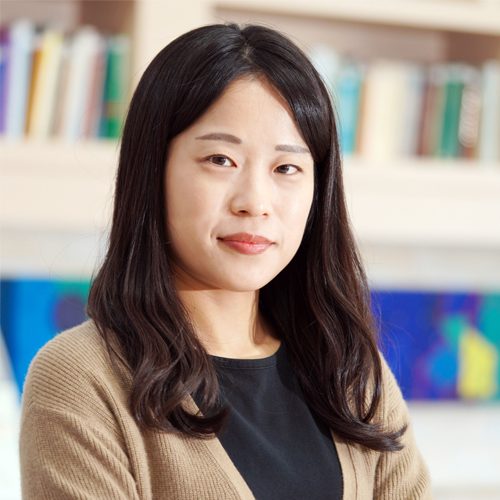
Research Highlight of Prof. Sunyoung Shin
In the era of artificial intelligence where big data can make significant contributions to science and society, we are inundated with numerous machine learning approaches and techniques. As a statistician and data scientist, I develop statistical models and methods that can draw novel scientific findings based on analysis and integration of big data. It is the key feature of the machine learning methods I have developed that their applications to data analysis are of scientific value with decent interpretations. The statistical learning and testing methods can deal with large-scale data and make interpretable learning possible in biomedical studies and genomics.
Efficient algorithms are designed for implementing the methodologies and distributed to the public to make impact to the academic community which needs such analytic resources. For rigorous support of the developed methodologies and algorithms, I am engaged in research on asymptotics and statistical theory. Novel computational and statistical methods that I develop are mainly used to address interesting inference and prediction problems in the fields such as genomics and epidemiology. The goal of genomic and epigenomic data analysis is to uncover underlying biological mechanisms; for example, identifying disease driver mutations and regulation of gene expression.
Here is the list of some research topics I have worked on:
i. development of novel parametric/semiparametric models and its inference for feature screening and structure recovery
ii. scalable test of statistical significance for DNA - transcription factor binding changes with genomic variants
iii. multi-view learning of omics and genomic data
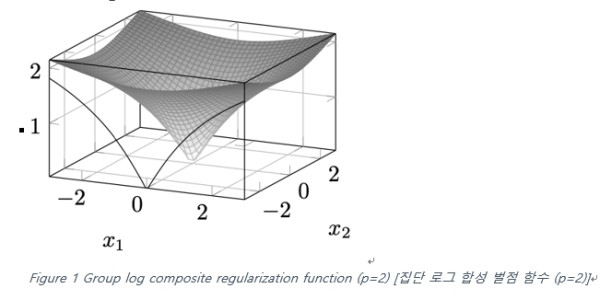
Selected publication
- Federico Abascal, Reyes Acosta, Nicholas J. Addleman et al. (2020). Expanded encyclopaedias of DNA elements in the human and mouse genomes. Nature, 583, 699-710. https://doi.org/10.1038/s41586-020-2493-4
- Federico Abascal, Reyes Acosta, Nicholas J. Addleman et al. (2020). Perspectives on ENCODE. Nature, 583, 693-698. https://doi.org/10.1038/s41586-020-2449-8
- Sunyoung Shin, Yufeng Liu, Stephen R. Cole, & Jason P. Fine. (2020). Ensemble estimation and variable selection with semiparametric regression models. Biometrika, 107(2), 433-448. https://doi.org/10.1093/biomet/asaa012
- Sunyoung Shin, Rebecca Hudson, Christopher Harrison, Mark Craven, & Sunduz Keles. (2019). atSNP Search: a web resource for statistically evaluating influence of human genetic variation on transcription factor binding. Bioinformatics, 35(15), 2657-2659. https://doi.org/10.1093/bioinformatics/bty1010
- Sunyoung Shin, & Sunduz Keles. (2017). Annotation regression for genome-wide association studies with an application to Psychiatric Genomics Consortium data. Statistics in Biosciences, 9(1), 50-72. https://doi.org/10.1007/s12561-016-9154-z
- Sunyoung Shin, Jason P. Fine, & Yufeng Liu. (2016). Adaptive estimation with partially overlapping models. Statistica Sinica, 26(1), 235-253. https://doi.org/10.5705/ss.2014.233